KEYNOTE
Title:
Business Process Mining meets Natural Language
Processing
Speaker:
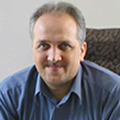
Prof. Mohsen Kahani Director of CERT and Web
Techology (WTLab) Labs,
professor of Computer engineering at Ferdowsi University of Mashhad, Iran
ABSTRACT:
Business process mining has gained much popularity recently, due
to its unique roles in discovery and monitoring business processes within
organizations. Process mining utilizes the real logs of enterprise software to
accomplish its tasks. However, these logs usually are either incomplete or
insufficient to provide enough feedback. Deploying other information such as
small text and description that accompany these logs can help further improve
the efficiency of process mining activities. In this talk, after introducing
process mining activities, the applications of natural language processing for
process mining are explored.
Home Page: http://kahani.profcms.um.ac.ir/
Title:
Computational Social Semantics for Mining Actionable Insights
Speaker:
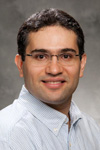
Dr. Ebrahim Bagheri Associate Professor in
the Department of Electrical and Computer Engineering at the Ryerson University
and the Canada Research Chair in Software and Semantic Computing, Senior Member
of the Institute of Electrical and Electronics Engineers (IEEE)
ABSTRACT:
Specificity is the level of detail at which a given term is
represented. Existing approaches to estimating term specificity are primarily
dependent on corpus-level frequency statistics. In this talk, I will discuss
how neural embeddings can be used to define corpus-independent specificity
metrics. Particularly, I will show how term specificity can be measured based
on the distribution of terms in the neighborhood of the given term in an
embedding space by leveraging geometric properties between embedded terms to
define various classes of specificity metrics. The talk will cover how such
specificity metrics can be useful in different information retrieval tasks such
as classifying terms into hierarchical categories, estimating query
performance, and ad hoc document ranking.
Home Page: https://www.ee.ryerson.ca/~bagheri/
Title:
A theory of anomaly detection in images
Speaker:
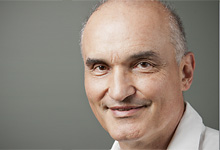
Dr. Jean-Michel Morel Ecole Normale Supérieure
Paris-Saclay, France
ABSTRACT:
Anomaly detection can not be formulated in a Bayesian framework: this
would require to simultaneously learn a model of the anomaly, and a model of
the background. (In the case where there are plenty of examples of the
background and for the object to be detected, neural networks may provide a
practical answer, but without explanatory power). In the case of
anomalies, we often dispose of only one image as unique informer on the
background, and of no example at all for the anomaly. Thus one is led to learn
a background model from very few samples, and to detect an anomaly as a large
deviation from this background model. I’ll show how the anomaly detection
problem can be led back to the simpler problem of detecting outliers in noise.
I’ll develop the proposed solution as a logical deduction of the huge
literature on anomaly detection. Work carried out in collaboration with
Axel Davy, Mauricio Delbracio and Thibaud Ehret.
Home Page: https://fr.wikipedia.org/wiki/Jean-Michel_Morel
|